Financial services companies have always walked a fine line between innovation and compliance. As AI adoption accelerates in the contact center space, a growing tension is surfacing—how do you gain the benefits of AI-driven Quality Assurance (Auto QA) without putting your customer data at risk?
The Problem with Cloud-Based Auto QA in Regulated Environments
Most Auto QA solutions today rely on the cloud. That means calls, transcripts, and metadata must leave a financial institution’s secure environment and travel to a third-party platform for analysis. Even with masking, redaction, and deletion protocols, sensitive data—like social security numbers, account details, and customer verification steps—are still exposed during transit.
For highly regulated sectors like banking, credit cards, and fintech, that creates friction. It’s not just a compliance checkbox, it’s a legal and operational risk that slows down adoption, drags InfoSec teams into prolonged evaluations, and complicates AI implementation.
Why That’s a Deal Breaker
Auto QA is only effective when it has access to the full customer interaction. But the very data AI needs to analyze, confirmation numbers, authentication steps, account activity, falls under strict PII protections. That puts security and compliance teams in a bind. Either they block access to this data and limit the effectiveness of AI scoring, or they take on significant risk by allowing sensitive data to flow into the cloud.
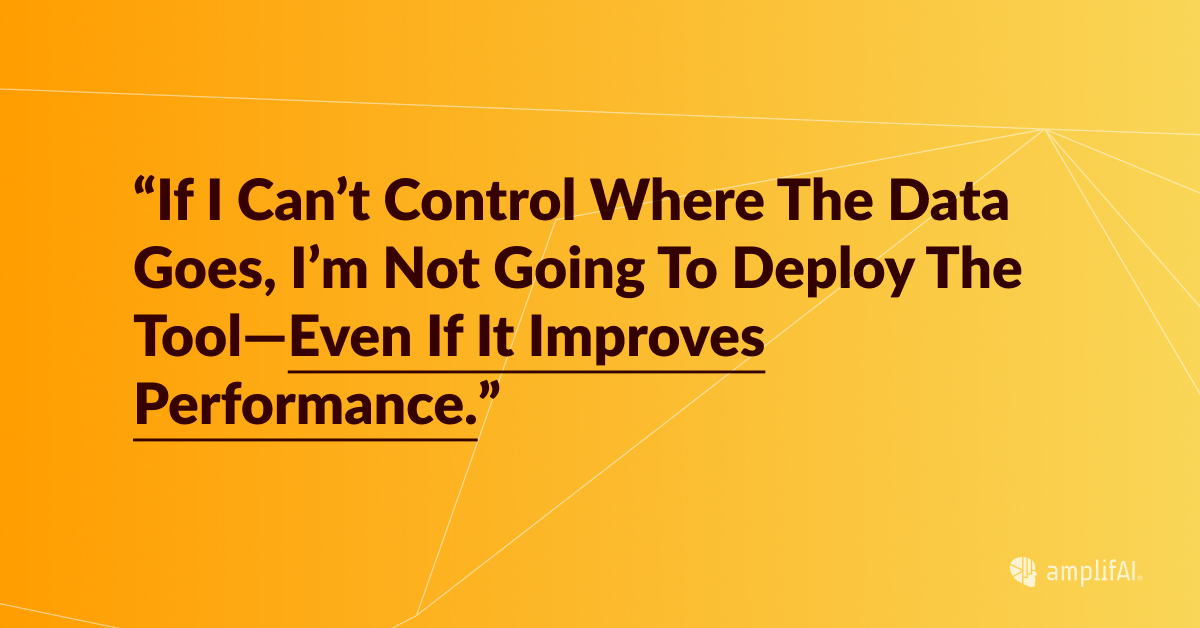
In many cases, they opt out altogether. As one financial services leader put it:
“If I can’t control where the data goes, I’m not going to deploy the tool, even if it improves performance.”
A Smarter Approach: Bring AI to the Data, Not the Other Way Around
The breakthrough isn’t in the algorithm—it’s in the architecture. Instead of sending sensitive customer interactions out to the cloud, leading-edge vendors are offering the ability to run the Auto QA engine inside the financial institution’s own data center.
This on-premise (or private cloud) model allows customer interactions to be transcribed, scored, and purged—without leaving the organization’s secure walls. The AI does its job locally: extracting sentiment, evaluating call flow adherence, and flagging coaching opportunities, all without ever touching a public cloud environment.
Advantages of On-Premise AI for Auto QA
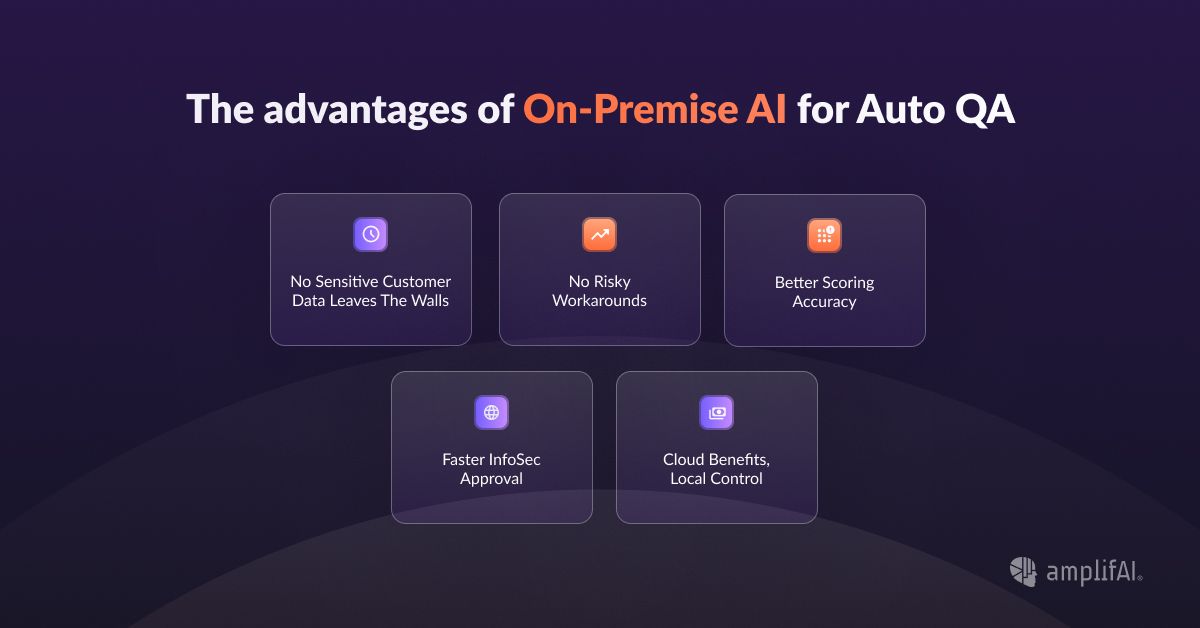
- No Sensitive Customer Data Leaves the Walls: All transcripts and audio can stay inside your network, fully under your control.
- No Risky Workarounds: No need to rely on masking or deletion promises. The data never leaves.
- Better Scoring Accuracy: Non-redacted transcripts allow for more complete, accurate AI evaluation.
- Faster InfoSec Approval: On-prem models streamline security reviews and reduce implementation time.
- Cloud Benefits, Local Control: You still get the benefits of AI, just deployed inside your infrastructure.
On-Premise AI-Powered Auto QA Isn’t Just for Banks
While this approach resonates most immediately with financial institutions, it applies to any industry handling sensitive data including, healthcare, insurance, government, and even large-scale retail or auto finance. The moment your calls include personal details, you need to ask: Can I afford for this data to leave the building?
Conclusion: AI Isn’t the Risk Your Architecture Is
Auto QA is a powerful lever for performance management. But for highly regulated environments, it has to be deployed in a way that respects data residency and ownership. By keeping AI inside the walls, you get the insights you need without exposing the data you can’t afford to lose.
At AmplifAI, we support both cloud and on-premise deployments of our AI-powered Auto QA engine. That means you get best-in-class performance management—delivered in the environment that makes the most sense for your security, compliance, and operations. Learn more about AmplifAI's Auto QA solution.