Call center analytics have become a foundational part of managing the customer experience in modern contact centers. As new technologies and communication channels expand the ways customers interact with brands, the volume and complexity of analytics data collected has grown dramatically.
Call center analytics are now foundational to managing customer experience in modern contact centers. As technology and communication channels have expanded, so has the complexity and volume of data generated by every customer interaction.
From voice calls and chats to CRM updates and post-call surveys, each touchpoint produces valuable data. While analytics tools have multiplied to meet the demand for deeper customer connection, the real challenge isn’t a lack of analytics, it’s having too much, and spread too thin.
Voice systems, QA platforms, CRM entries, channel metrics, homegrown reports, sentiment trackers—each system generates insights. The result? Most contact center teams are drowning in dashboards.
.png)
Contact center leaders report spending up to 40% of their day just reviewing analytics data across disconnected dashboards. Agents end up hit with coaching feedback they can’t contextualize, or none at all.
Instead of driving improvement, extracting insights from analytics data has become an operational burden at every level of the organization.
In this guide we're breaking down the core types of call center analytics, how to interpret them, and the operational risks of relying on fragmented or manual data analysis. We’ll also explore how AI-powered call center analytics software is shifting this model from analysis and report review to real-time intelligence and action.
Topics we're covering:
- What are call center analytics
- Call center analytics data sources
- Types of call center analytics data
- Challenges of call center analytics
- Consequences of poor call center analytics data management
- How to analyze contact center analytics
- How AI optimizes your call center analytics data
- Call center analytics software
- Operationalize your call center analytics
- Call center analytics FAQ's
Looking for AI software to operationalize your call center analytics?
Don't miss our section on operationalizing your call center analytics with software.
What are Call Center Analytics?
Call center analytics is the practice of interpreting data from customer interactions to uncover insights into agent performance, customer satisfaction, call trends, and service adherence.
Call center analytics primarily aims to unveil actionable insights from agent interactions that drive better decision-making, deeper comprehension of customer needs, and improved business processes through data-driven problem-solving.
So where does this data come from? Let’s break down the core data sources powering modern call center analytics.
Call Center Analytics Data Sources
Below are key data sources commonly used in call center analytics. Each provides unique insights into agent performance, customer sentiment, and operational bottlenecks.
What's the Difference Between Call Center Analytics and Call Center Metrics (KPIs)?
It’s a common misconception that call center analytics and KPIs are the same. In reality, they serve distinct roles in performance management.
Call center analytics focuses on data inputs, the raw signals from interactions, behaviors, and systems. In contrast, call center productivity KPIs represent outputs, measurable results like average handle time (AHT), call volume, or customer satisfaction scores.
Think of analytics as the engine that interprets behavior, while KPIs are the dashboard dials that reflect what’s happening. Metrics like average hold time, first call resolution, and net promoter score (NPS) are derived from analytics, but don’t explain why those results occur.
Without robust call center analytics, productivity KPIs lack the context needed to drive action. The data sources behind call center analytics are the foundation for any accurate, meaningful performance metric.
Types of Call Center Analytics
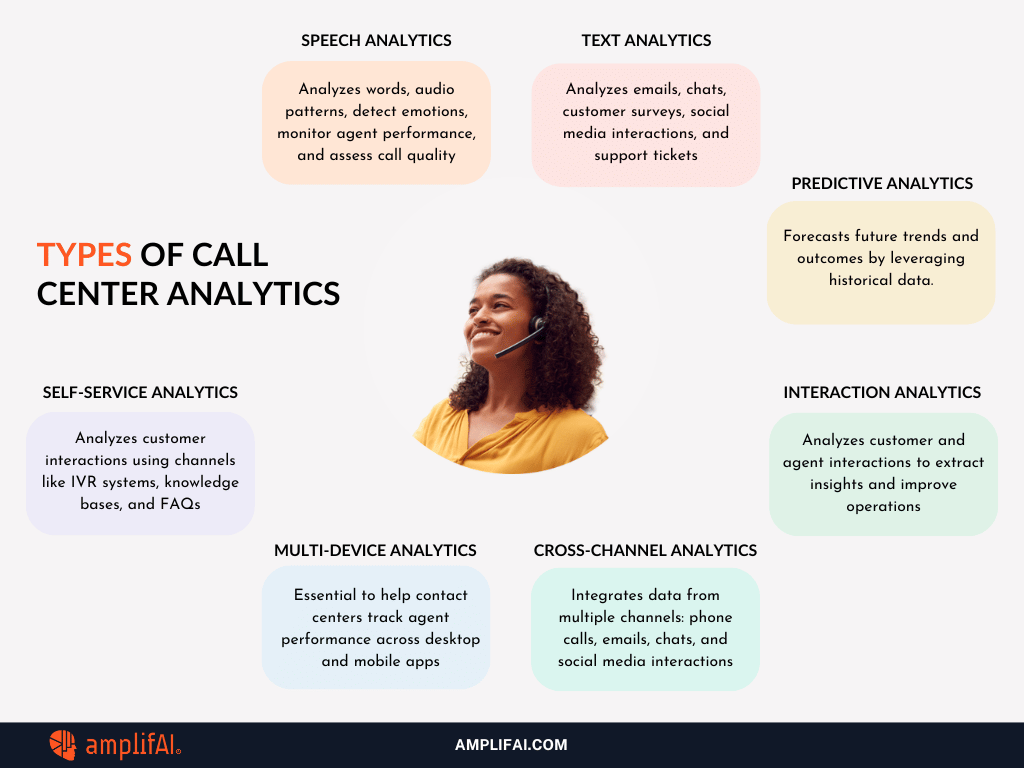
Each type of call center analytics data provides unique insights into different aspects of call center operations, customer interactions, and team performance. By leveraging these analytics effectively, you can improve efficiency, bolster customer satisfaction, and drive overall business success in your contact center.
Here's a quick summary of the 7 most important types of call center analytics. Click any type to jump to the full breakdown below:
Speech analytics involves leveraging AI to analyze recorded calls to extract valuable insights, including identifying words, analyzing audio patterns to detect emotions, monitoring agent performance, and assessing call quality.
According to McKinsey,
Speech analytics implementation results in 20-30% cost savings and a 10% improvement in customer satisfaction scores.
Speech analytics comprises of:
With speech analytics, you can use the large number of conversations being stored and transform those into valuable insights that will benefit businesses by helping them discover trends, customer preferences, and opportunities for process improvement or training.
When analyzed correctly, speech analytics:
Learn more about speech analytics in call centers.
Text analytics analyzes written communications such as emails, chats, customer surveys, activity in your interactive catalog, social media interactions, and support tickets to extract valuable insights and improve call center operations.
Text analytics provide insights into customer preferences, common issues, and opportunities for improving written communication strategies.
Here's how text analytics works and its applications:
Predictive analytics helps you forecast future trends and outcomes by leveraging historical data. In call centers, predictive analytics can forecast call volumes, staffing requirements, customer behavior, and potential issues.
Contact center analytics uses AI to analyze call center data and discover future trends and patterns to address or ignore potential issues.
Call centers can proactively adjust staffing levels, resources, and processes by anticipating future trends to meet demand and improve efficiency.
Predictive analytics applications:
Predictive analytics empowers call centers to make data-driven decisions, anticipate customer needs, optimize operations, and enhance customer experience.
Call center interaction analytics analyzes customer and agent interactions to extract insights and improve operations.
Contact centers can use AI, machine learning, natural language processing, and neural networks to identify customer preferences and pain points.
Interaction analytics allows you to recognize recurring issues or dissatisfaction areas and work on improving overall customer satisfaction. It helps improve first call resolution (FCR) by identifying factors leading to repeated calls for the same issue, which enhances FCR rates.
Desktop analytics track the performance and movement of contact center agents using desktop computers. Meanwhile, mobile analytics monitors mobile devices, such as tablets and smartphones.
Together, desktop and mobile analytics help you address slow bandwidth and security weaknesses. Teams can also monitor call center agents' apps and tools and whether they use them correctly.
They also help with real-time call monitoring by capturing inefficiencies, enhancing security, and identifying coaching opportunities for phone agents. This analysis can uncover redundant tasks, reduce call handling time, and improve overall efficiency, benefiting agents and customers.
Cross-channel analytics integrates data from multiple communication channels, such as phone calls, emails, chats, and social media interactions, giving a comprehensive view of customer calls and behavior across all touchpoints.
A call center must identify its customers' preferred channels and customize service accordingly. This helps them equip phone agents with this data, allowing personalized interactions.
For instance, if a customer primarily conducts banking online, agents can be alerted in real-time to offer online solutions. Just as with speech analytics, this area is emerging but likely to become indispensable in the future.
Cross-channel analytics help identify customer preferences, trends, and issues that span multiple channels, enabling call centers to deliver a seamless omnichannel experience.
Self-service analytics analyzes customer interactions using channels like IVR systems, knowledge bases, and FAQs.
Contact centers are now exploring methods to encourage self-service channels. For instance, if customers contact a call center agent to update their address, why not provide an online option on your website?
This helps minimize errors, decreases incoming call volumes, and reduces company costs.
Self-service analytics help optimize self-service channels, improve usability, and reduce call volumes by empowering customers to find answers and resolve issues independently.
Challenges of Call Center Analytics
Sometimes, too much of a good thing can become overwhelming, especially when it's not utilized effectively. This is the paradox of call center analytics: there's an abundance of data but too little time and/or too few resources to make sense of it and turn it into actual and measurable performance indicators.
.png)
Disjointed and siloed contact center analytics data hinders the effectiveness of call center operations and overall performance.
1. Overwhelming Volume of Data
Contact centers capture massive amounts of data from calls, chats, emails, and more. But without structure or prioritization, this volume burdens teams instead of guiding them.
2. Unstructured or Inaccurate Data
Inconsistent formats, duplicated records, and missing context across systems lead to poor insights. Dirty data makes confident decision-making nearly impossible.
3. Siloed Systems and Tools
Data stored across disconnected platforms (QA, CRM, WFM, custom tools) is hard to reconcile. Without integration, analytics remain partial and under-utilized.
4. Skill Gaps in Data Interpretation
Frontline managers and agents aren’t always trained to translate analytics into performance improvements. Without the right tools or context, the data stays dormant.
5. Manual and Time-Consuming Processes
Manual reporting takes hours, delays feedback loops, and invites error. As call centers grow, manual approaches can’t scale with the complexity of operations.
Consequences of Poor Call Center Analytics Management
When the wealth of data available isn't harnessed correctly, the fallout extends far beyond inefficiency.
1. Inefficient Resource Allocation
Without actionable insights, teams misallocate resources, understaffing key channels, overburdening agents, or overinvesting in low-impact areas.
2. Reactive Problem-Solving
Managers are forced to rely on instinct instead of insight, addressing issues only after they’ve escalated, rather than preventing them with proactive analytics.
3. Missed Opportunities for Improvement
Without clear visibility into agent performance, customer behavior, or process breakdowns, contact centers miss the data cues that could drive smarter coaching, better outcomes, and faster improvements.
4. Stagnant Performance and Competitive Decline
Inconsistent analytics usage leads to plateaus in productivity, slower decision-making, and an inability to adapt to evolving customer expectations or market shifts.
How to Manually Analyze Call Center Analytics
.png)
Following the 8 steps below, you can analyze your call center analytics data to drive continuous improvement and deliver better customer experiences.
8 Steps to Analyze Contact Center Analytics
How AI Turns Call Center Analytics into Real-Time Operational Impact
We've established that managing the vast amounts of data and analytics generated daily in call centers can lead to missed opportunities and financial losses.
But with the right application of AI, this flood of data can be transformed into a powerful asset, boosting overall call center productivity and service delivery.
Here are some examples of how contact centers leverage analytics and AI to optimize operations:
- Get actionable AI-powered insights
- Predict call load and agent’s performance
- Enhance customer satisfaction
- Boost automation and efficiency
- Provide the right coaching opportunities
Call Center Analytics Software
Call center analytics don’t drive performance, actions do.
That’s why AmplifAI doesn’t just show you dashboards. It connects the dots between data, performance, and action, unifying all types of call center analytics insights across speech, QA, CRM, surveys, spreadsheets, and even homegrown systems into one operational layer built for action.
With 150+ native integrations and a continuously updating data hub, AmplifAI eliminates silos and manual reporting. Every stakeholder from CX leaders, supervisors, QA teams, and agents gets real-time, role-specific insight where it counts most.
How AmplifAI Unifies and Uses Your Call Center Analytics
Most platforms aggregate data. AmplifAI goes further structuring it into an AI-ready foundation that powers performance workflows, auto QA, coaching, and GenAI.
By transforming fragmented analytics into unified, actionable intelligence, AmplifAI helps contact centers shift from post-mortem reviews to real-time decision-making.

1. AI-Ready Data Integration Across Every System
AmplifAI's patented call center data integration process connects across cloud, hybrid, or on-prem environments, ingesting and continuously updating all your call center analytics data from CRMs, call recordings, IVRs, surveys, and custom systems, without requiring a heavy IT lift.
All your call center analytics data, unified into one operational intelligence engine.
.png)
2. Role Based Performance Management Dashboards
AmplifAI's call center performance management software with role-based performance management dashboards give each stakeholder, supervisors, QA, and agents a real-time view tailored to their goals. Unlike other call center performance management tools that claim to unify data, AmplifAI takes call center analytics and turns them into performance management powerhouses.

Every role sees what matters most:
- Supervisors get next-best coaching actions
- QA sees automated scoring + high-risk patterns
- Agents receive real-time feedback and recognition
3. Data-Driven Coaching

AmplifAI's call center coaching software with AI-enabled coaching uses call center analytics data to surface high-performer behaviors, identify skill gaps, and deliver targeted coachings for team leaders to act on, instantly. Unlike other call center coaching tools where coaching, performance management, QA, and CX Insights are siloed, with AmplifAI everything is connected, coaching becomes personalized, automated, and outcome-driven.
4. Agent Engagement Through Recognition & Gamification

Turn insights into impact. AmplifAI transforms performance data into real-time gamification and recognition, badges, and gamified goals, boosting morale, retention, and agent productivity.
5. Automated Quality Assurance & Compliance

AmplifAI’s call center quality assurance software, including AI-powered Auto QA, connects QA analytics data directly to coaching workflows and performance metrics dashboards. Unlike other call center QA tools, AmplifAI named a leading automated quality assurance software by the CMP Prism report transforms hours of manual QA analytics review into real-time, trackable insights that improve outcomes and ensure compliance.
Quality assurance and performance management are no longer siloed, AmplifAI unifies them through intelligent automation.
.png)
AmplifAI was named a Leading Automated QA and Quality Management Software provider in the 2025 CMP Research Prism an independent evaluation of 17 contact center platforms based on innovation, implementation, and customer impact.
The report highlights key investment criteria across automation, coaching integration, and real-time performance outcomes. AmplifAI’s inclusion reinforces its position as a unified platform for QA, analytics, and coaching, delivering measurable improvements across compliance, quality, and agent performance.
Conclusion
Call center analytics shouldn't just report on the past, it should drive the future.
When unified and powered by AI, call center analytics go from being an operational burden to a real-time AI-powered decision engine that fuels coaching, QA, performance management, and customer experience all from a single, actionable layer.
AmplifAI Contact Center AI platform transforms call center analytics into measurable outcomes across efficiency, service quality, and agent performance without adding burden to your leaders or IT teams.
See how forward-thinking contact centers are using AmplifAI to operationalize call center analytics turning data into action.
Operationalize Your Call Center Analytics
If you’re ready to operationalize your call center analytics, these expert-reviewed guides will help you implement the right tools for the job.
👉 Explore the Best Call Center Performance Management Software (AmplifAI unifies metrics, coaching, and QA into actionable dashboards).
👉 See the Top Call Center Coaching Software Platforms (AmplifAI ranks #1 for coaching effectiveness and next-best action workflows).
👉 Discover the Best Call Center Quality Assurance Software (AmplifAI automates QA scoring and links feedback to coaching in real time).
👉 Read the Best Call Center Gamification Software Guide (AmplifAI uses analytics data to boosts agent engagement through real-time recognition + performance rewards).
Call Center Analytics FAQs
What is call center analytics used for?
Call center analytics help teams uncover trends, agent behavior patterns, customer sentiment, and system performance. The goal is to turn interaction data into insights that drive better decisions, coaching, and customer experiences.
👉 Learn how to manually analyze call center analytics.
How does call center analytics improve customer experience?
By analyzing interactions, call center analytics reveals trends and sentiment, enabling personalized service, faster resolutions, and proactive issue prevention.
👉 See how call center analytics impact CX.
How does AI improve call center analytics?
AI transforms raw data into real-time insights, predicts outcomes, automates analysis, and personalizes agent coaching at scale. Learn more how AI transforms raw data into real-time coaching signals.
👉 Read more about how AI improves call center analytics.
What does call center analytics measure?
Call center analytics measures agent behavior, customer sentiment, call trends, channel usage, and self-service effectiveness using voice, text, and interaction data.
Read more on the explore all 7 types of call center analytics.
What types of data are used in call center analytics?
Call center analytics pulls from sources like voice transcriptions, CRM systems, chat logs, surveys, and IVR interactions, each offering different insights into customer behavior and agent performance.
View the full list of call center analytics data sources
What makes AmplifAI Call Center Analytics Software different?
AmplifAI's call center data integration process ingests and manages all types of call center analytics into an AI-ready continuously updating layer that transforms analytics into actions. Rather than creating new work for CX leaders and contact center teams, AmplifAI removes the burden of data analysis by sending it to role-based performance dashboards for every level of the org from agents to executives and everyone in-between.